Predicting Poor Outcomes Among Individuals Seeking Care for Major Depressive Disorder
Abstract
Objective
To develop and validate algorithms to identify individuals with major depressive disorder (MDD) at elevated risk for suicidality or for an acute care event.
Methods
We conducted a retrospective cohort analysis among adults with MDD diagnosed between January 1, 2018 and February 28, 2019. Generalized estimating equation models were developed to predict emergency department (ED) visit, inpatient hospitalization, acute care visit (ED or inpatient), partial‐day hospitalization, and suicidality in the year following diagnosis. Outcomes (per 1000 patients per month, PkPPM) were categorized as all‐cause, psychiatric, or MDD‐specific and combined into composite measures. Predictors included demographics, medical and pharmacy utilization, social determinants of health, and comorbid diagnoses as well as features indicative of clinically relevant changes in psychiatric health. Models were trained on data from 1.7M individuals, with sensitivity, positive predictive value, and area‐under‐the‐curve (AUC) derived from a validation dataset of 0.7M.
Results
Event rates were 124.0 PkPPM (any outcome), 21.2 PkPPM (psychiatric utilization), and 7.6 PkPPM (suicidality). Among the composite models, the model predicting suicidality had the highest AUC (0.916) followed by any psychiatric acute care visit (0.891) and all‐cause ED visit (0.790). Event‐specific models all achieved an AUC >0.87, with the highest AUC noted for partial‐day hospitalization (AUC = 0.938). Select predictors of all three outcomes included younger age, Medicaid insurance, past psychiatric ED visits, past suicidal ideation, and alcohol use disorder diagnoses, among others.
Conclusions
Analytical models derived from clinically‐relevant features identify individuals with MDD at risk for poor outcomes and can be a practical tool for health care organizations to divert high‐risk populations into comprehensive care models.
HIGHLIGHTS
The predictive models developed in this study accurately identified individuals at near‐term risk for suicidal ideation, suicide attempt, psychiatric inpatient hospitalization, and psychiatric emergency department visit.
Analytical models can provide a flexible and practical tool for health care organizations to divert high‐risk populations into comprehensive care models.
These, or related, algorithms should be automated and integrated into systems with administrative claims data or modified for use in E.H.R. systems to guide health systems, care providers, or depression care management program in routing individuals with major depressive disorder to the most appropriate types of care at the appropriate frequencies to adequately manage their disease.
Major depressive disorder (MDD) is a common, chronic, episodic condition with symptoms that affect how an individual feels, thinks, and acts (1). MDD can adversely impact the ability to function, to work, to participate in school, and to enjoy life (2, 3, 4, 5, 6). A severe depressive episode can result in suicidal ideation or attempts (7) and lead to a need for an acute healthcare intervention (8, 9, 10).
Primary care is the dominant practice setting for individuals seeking MDD care (11). Yet, without a team‐based approach, an individual primary care provider can struggle to deliver consistent, high‐quality behavioral health care—especially to individuals struggling with chronic or more severe depression (12, 13). Decades of research confirm that a collaborative care model, a team‐based approach that integrates behavioral healthcare providers into a primary care setting, is significantly more effective than a model that relies on management by an individual provider or by referral to specialty care (14, 15).
Collaborative care models, however, can be challenging and costly to implement (16, 17). Multidisciplinary care models may be more cost‐effective if offered to priority patients, specifically those who are at high risk of poor outcomes. To do this, healthcare providers need a valid, practical way to identify at‐risk individuals. Recently, the application of advanced analytics to available digital healthcare data has highlighted the precision of models that predict suicidality using structured data (18, 19) and unstructured data, applying natural language processing techniques (20). Further, numerous studies have used administrative healthcare data to predict hospitalization in general populations (21, 22, 23) and among individuals with comorbid depressive symptoms (24, 25).
The objective of this study was to develop and validate, in a U.S. national population of community‐dwelling adults, a comprehensive set of analytical models to predict both suicidality and the use of acute care services among individuals with MDD.
METHODS
This study was a retrospective cohort analysis of individuals 18 years or older diagnosed with MDD between March 1, 2018, and February 28, 2019. The study received an exempt determination from the Advarra Institutional Review Board.
Data Sources
Individuals were identified from healthcare insurance claims data licensed from Clarivate Real‐World Data (https://clarivate.com/products/real‐world‐data/?lid=d). This dataset, which does not include benefits eligibility information, includes medical and pharmacy claims from an estimated 220 million individuals. Social determinants of health were measured at the county level from the Area Health Resources File available from the Department of Health and Human Services (www.data.hrsa.gov/topics/health‐workforce/ahrf). Each eligible individual was assigned to a county using their 3‐digit ZIP code of residence linked to the 5‐digit ZIP code associated with their primary care or mental health care provider.
Study Periods and Events
The case finding period was March 1, 2018, through February 28, 2019. The index date was the date of the first qualifying claim associated with MDD during the case finding period. The outcome event period was the 12 months following the index date. Risk factors were identified in the 12‐months preceding index (baseline) and monthly throughout the outcome event period.
Identification and Selection of Study Participants
Eligible study participants had two or more outpatient claims associated with a diagnosis code of MDD (ICD‐10: F32.0–F32.9; F33.0–F33.9) at least 30 days or more apart or one inpatient claim with MDD in the primary position during the case‐finding period, were 18+ years of age at the beginning of baseline, and had one medical or pharmacy claim in each 3‐month period from baseline through follow up.
Predictors
Participant‐specific age, sex, region of residence, and insurance provider (Medicaid, Medicare, commercial, or VA/other) were defined from claims data. Social determinants of health characteristics were measured at the county level and included percent graduated high school, unemployment rate, percent impoverished, percent of population by racial and ethnic groups, population density, primary care provider density, mental health provider density, and median household income. Comorbidities were identified in each month to reflect changing risk within patients over time. Comorbidities included psychiatric comorbidities (attention deficit disorder, schizophrenia, substance use disorder, alcohol use disorder, anxiety, autism, dementia, eating disorder, personality disorder, post‐traumatic stress disorder [PTSD], psychosis, delirium, other psychotic disorder); medical comorbidities (hypertension, myocardial infarction, congestive heart failure, peripheral vascular disease, dementia, cerebrovascular disease, chronic pulmonary disease, rheumatic disease, peptic ulcer disease, diabetes, liver disease, hemiplegia paraplegia, renal disease, cancer, and AIDS); and injury/poisoning diagnoses (traumatic brain injury, injury/poisoning, suicidal ideation, suicidal behavior, and self‐inflicted laceration).
Incident diagnoses were identified by the presence of an ICD‐10 code during the outcome events period and defined by a primary position ICD‐10 code on a medical claim for a disorder that was not included in any position on a baseline medical claim. Incident disorders included substance use disorder, alcohol use disorder, self‐harm, psychosis, mood disorder, personality disorder, PTSD, adjustment disorder, eating disorder, anxiety disorder, and injury/poisoning. MDD severity and changes in severity were defined by ICD‐10 codes. Examples of changes in severity include an ICD‐10 code for F32.1 (single episode, moderate severity) following a previous code of F32.0 (single episode, mild severity) in the prior month; a code indicating psychotic features following a code not including psychotic features; and a change in codes indicating a transition from full or partial remission to a code without reference to remission.
Counts of past healthcare service use and change in counts from the prior month were also included. Past service use included emergency department (ED) visits (psychiatric and non‐psychiatric), inpatient admissions (non‐psychiatric, non‐MDD psychiatric, MDD‐related), 30‐day all‐cause readmissions, outpatient follow‐up care within 7 days of any psychiatric admission, electroconvulsive therapy procedures, transcranial magnetic stimulation (TMS) procedures, visits to psychiatrists, visits to primary care physicians, psychotherapy sessions, and use of prescription medications (antidepressants, antipsychotics, anticonvulsants, anxiolytics, sedatives, hypnotics).
Indicators for changes in medications within each month and between months were developed, including whether a new medication was added, and whether that drug was from a new therapeutic class or a different active ingredient within the same class, whether the dose increased or decreased, whether the medication was removed, and a continuous measure of adherence which was defined as the number of days' worth of medication that was filled minus the number of days between filled prescriptions.
Outcome Events
Outcomes included two major event categories: acute care services and suicidality. Acute care services included all‐cause ED visits, psychiatric ED visits, MDD‐specific short‐term inpatient hospitalizations, MDD‐specific inpatient psychiatric facility admissions, and MDD‐specific partial‐day hospitalizations. Suicidality included suicidal ideation and suicidal behavior (attempt). Each outcome event was dichotomized (yes, if event occurred) and calculated in each month of the 12‐month outcome events period for each individual in the study.
Statistical Analysis
Baseline demographic, comorbidity, and treatment use characteristics were compared between the training and validation samples using chi‐squared tests of statistical significance. Outcomes were reported per 1000 patients per month (PkPPM). Time to first outcome was reported using means, standard deviations, and medians and depicted graphically with Kaplan Meier plots.
To address the issue of low outcome event rates in each outcome measure except all‐cause ED visits, Synthetic Minority Over‐Sampling Technique (SMOTE), which generates new observations of the minority class based on nearest neighbors determined by Euclidean distance in feature space (26), was applied to all outcomes except all‐cause ED visits which did not have a data imbalance issue. Outcome event rates ranged from 40% to 50% after oversampling.
For the prediction models, the unit of analysis was patient‐month defined as consecutive 30‐day periods beginning from the MDD diagnosis index date. During each patient‐month, the presence/absence and count of predictors within the month, and changes from the prior month were measured. The 2,386,348 eligible individuals were divided randomly, 70% into a training set (n = 1,671,835) and 30% (n = 714,513) into a validation set. The training set was used to build and train the initial predictive model and the validation set was used to evaluate the performance of training models in a different population with similar characteristics as well as to assess overfitting during the training phase. To identify predictive factors, all potential predictors were included in multivariate regression models as independent variables with events serving as the outcomes. The outcomes were dichotomized and calculated in each patient‐month of the outcome events period. Because the same patient could have several exposure and outcome events, and to reflect changes in risk within patients over time, generalized estimating equations were used to account for within‐patient correlation. Separate models were estimated for the following outcomes: all‐cause ED visits, psychiatric ED visits, MDD‐specific short‐term inpatient hospitalizations, MDD‐specific inpatient psychiatric facility admissions, MDD‐specific partial‐day hospitalizations, suicidal ideation, suicidal behavior (self‐harm attempt), and composite measures for any psychiatric acute care service, suicidality (ideation or self‐harm event). All models predicted outcomes occurring in the following 30 days. The initial multivariate generalized estimating equation model included all potential predictors. Using backward elimination, less strongly associated variables were removed from the model one at a time until all predictors were significant at the p < 0.01 level. The training dataset was used to develop the predictive models, after which the models were applied to the validation set to obtain predicted probabilities and the following model performance statistics: area‐under‐the‐curve (AUC), sensitivity, specificity, and positive predictive value (PPV).
Analyses were conducted using SAS version 9.4 (SAS Institute, Inc.) and R version 4.1.1 (The R Foundation).
RESULTS
The total eligible study population included 2,386,348 individuals. The average age was 58.8 years (SD: 17.3 years), most individuals were female (70.7%), and included similar proportions of individuals insured by Medicaid (34.0%), Medicare (33.1%), and commercial plans (32.6%). Comprising 41.1% of the population, comorbid anxiety was common as was substance use disorder (20.4%). The overall Charlson Comorbidity Index was 1.9 (SD: 2.2). Approximately 1 in 3 individuals had a psychiatry visit and 1 in 5 individuals had a psychotherapy session during the baseline period. Selective serotonin reuptake inhibitors (36.6%) were the most prescribed antidepressant class, followed by serotonin‐norepinephrine reuptake inhibitors (19.1%) and bupropion (14.0%).
In the year following an MDD diagnosis, 43.9% of the population had at least one ED visit, 8.2% had one or more acute care visits attributed to a psychiatric cause, and 3.8% had documented suicidality, with 3.2% having one or more suicidal ideation events and 1.2% having at least one documented self‐harm event (Table 1). Of the psychiatric acute care service visits, the most common was a psychiatric ED visit (4.2%) followed by an inpatient hospitalization for MDD (3.3%), inpatient hospitalization at a psychiatric facility (0.7%) and a partial‐day hospitalization (0.2%).
Total population | Training sample | Validation sample | p‐value | ||||
---|---|---|---|---|---|---|---|
2,386,348 | 1,671,835 | 714,513 | |||||
n | % | n | % | n | % | ||
Age | |||||||
18–30 | 191,224 | 8.0% | 133,741 | 8.0% | 57,483 | 8.0% | NS |
31–49 | 479,583 | 20.1% | 335,743 | 20.1% | 143,840 | 20.1% | |
50–64 | 722,553 | 30.3% | 506,131 | 30.3% | 216,422 | 30.3% | |
Over 65 | 992,975 | 41.6% | 696,208 | 41.6% | 296,767 | 41.5% | |
Sex | |||||||
Female | 1,687,948 | 70.7% | 1,182,235 | 70.7% | 505,713 | 70.8% | NS |
Male | 698,400 | 29.3% | 489,600 | 29.3% | 208,800 | 29.2% | |
Insurance | |||||||
Medicaid | 811,115 | 34.0% | 568,751 | 34.0% | 242,364 | 33.9% | NS |
Medicare | 789,982 | 33.1% | 553,178 | 33.1% | 236,804 | 33.1% | |
Commercial | 777,404 | 32.6% | 544,381 | 32.6% | 233,023 | 32.6% | |
VA/other | 7847 | 0.3% | 5525 | 0.3% | 2322 | 0.3% | |
Comorbidities | |||||||
Anxiety | 981,908 | 41.1% | 687,690 | 41.1% | 294,218 | 41.2% | NS |
Substance use disorder | 487,283 | 20.4% | 341,224 | 20.4% | 146,059 | 20.4% | NS |
Alcohol use disorder | 136,750 | 5.7% | 95,889 | 5.7% | 40,861 | 5.7% | NS |
Treatment (baseline) | |||||||
Psychiatry (yes/no) | 779,056 | 32.6% | 545,002 | 32.6% | 234,054 | 32.8% | <0.05 |
Psychotherapy (yes/no) | 485,293 | 20.3% | 340,218 | 20.3% | 145,075 | 20.3% | NS |
SSRIs | 872,789 | 36.6% | 610,905 | 36.5% | 261,884 | 36.7% | <0.05 |
SNRIs | 456,226 | 19.1% | 319,486 | 19.1% | 136,740 | 19.1% | NS |
Bupropion | 333,756 | 14.0% | 233,730 | 14.0% | 100,026 | 14.0% | NS |
Tricyclics and tetracyclics | 194,285 | 8.1% | 136,272 | 8.2% | 58,013 | 8.1% | <0.05 |
Serotonin modulators | 80,656 | 3.4% | 56,363 | 3.4% | 24,293 | 3.4% | NS |
Alpha‐2s | 168,861 | 7.1% | 118,394 | 7.1% | 50,467 | 7.1% | NS |
Anxiolytics | 388,376 | 16.3% | 271,860 | 16.3% | 116,516 | 16.3% | NS |
Antipsychotics | 224,536 | 9.4% | 157,188 | 9.4% | 67,348 | 9.4% | NS |
ECT | 5470 | 0.2% | 3849 | 0.2% | 1621 | 0.2% | NS |
TMS | 3391 | 0.1% | 2338 | 0.1% | 1053 | 0.1% | NS |
Outcomes | |||||||
ED visits (all‐cause) | 1,047,139 | 43.9% | 733,198 | 43.9% | 313,941 | 43.9% | NS |
Acute care, psych (overall) | 195,237 | 8.2% | 136,618 | 8.2% | 58,619 | 8.2% | NS |
ED visit (psych) | 99,148 | 4.2% | 69,549 | 4.2% | 29,599 | 4.1% | NS |
Inpt hospitalization (MDD) | 78,665 | 3.3% | 55,050 | 3.3% | 23,615 | 3.3% | NS |
Inpt psych facility (MDD) | 17,878 | 0.7% | 12,570 | 0.8% | 5308 | 0.7% | NS |
Partial‐day hospitalization | 4547 | 0.2% | 3165 | 0.2% | 1382 | 0.2% | NS |
Suicidality (overall) | 90,250 | 3.8% | 63,057 | 3.8% | 27,193 | 3.8% | NS |
Suicidal ideation | 75,191 | 3.2% | 52,502 | 3.1% | 22,689 | 3.2% | NS |
Self‐harm attempt | 28,213 | 1.2% | 19,714 | 1.2% | 8499 | 1.2% | NS |
The distribution of demographic, comorbidity, treatment, and outcome events were comparable among individuals assigned to the training and validation population subgroups. The statistically significant differences reflect the large sample size and do not appear to reflect meaningful differences between the groups (Table 1).
The suicidality event rate overall was 7.6 events per thousand patients per month (PkPPM), with suicidal ideation reported at 6.0 events PkPPM and self‐harm attempts at 1.6 PkPPM (Figure 1). The overall rate of psychiatric‐specific service utilization was 21.2 PkPPM, with the highest rate noted for psychiatric ED visits (8.0 PkPPM) followed by MDD‐specific inpatient hospitalizations (5.1 PkPPM), MDD‐specific inpatient admission to a psychiatric facility (1.4 PkPPM), and partial‐day hospitalizations (0.7 PkPPM). The all‐cause ED rate was the most frequent event at 114.3 visits PkPPM (Figure 1).

FIGURE 1. Time‐to‐event for acute care services or suicidality among individuals in the validation sample.
Individuals with outcome events in the follow‐up period varied significantly from individuals with no outcomes on nearly all predictive characteristics (Table 2). Compared to individuals with no outcomes in the follow‐up period, individuals with a suicidality event were significantly younger (49.8 vs. 59.5 years; p < 0.01), more likely male (33.7% vs. 28.5%; p < 0.01) and diagnosed with anxiety (53.2% vs. 39.8%; p < 0.01), substance use disorder (38.7% vs. 18.2%; p < 0.01), or alcohol use disorder (13.4% vs. 4.3%; p < 0.01). In addition, these individuals were more likely to be receiving psychiatric care prior to the index diagnosis date, including receiving any psychiatry care (40.9% vs. 26.6%; p < 0.01), psychotherapy (25.7% vs. 18.2%; p < 0.01), and/or antipsychotic pharmacotherapy (18.3% vs. 7.7%; p < 0.01). Though elevated, only 18.1% and 8.9% of those with a suicidality event in the follow‐up period had ideation or a self‐harm attempt, respectively, in the baseline period. Both rates are significantly higher than those without suicidality in the follow‐up period. Similar patterns are noted for individuals with one or more acute psychiatric care outcomes in the follow‐up period.
None | Psychiatric ED/hospitalization | Suicidality | Both | p‐value | |||||
---|---|---|---|---|---|---|---|---|---|
655,894 | 31,426 | 6258 | 20,935 | ||||||
n | % | n | % | n | % | n | % | ||
Age, years (mean/SD) | 59.5 | 17.1 | 53.3 | 17.6 | 49.8 | 18.1 | 46.1 | 16.2 | <0.01 |
Sex | |||||||||
Female | 468,863 | 71.5% | 20,636 | 65.7% | 4147 | 66.3% | 12,067 | 57.6% | <0.01 |
Male | 186,969 | 28.5% | 10,790 | 34.3% | 2111 | 33.7% | 8868 | 42.4% | <0.01 |
Comorbidities | |||||||||
Anxiety | 260,870 | 39.8% | 17,289 | 55.0% | 3328 | 53.2% | 12,731 | 60.8% | <0.01 |
Substance use disorder | 119,628 | 18.2% | 12,390 | 39.4% | 2419 | 38.7% | 11,622 | 55.5% | <0.01 |
Alcohol use disorder | 28,444 | 4.3% | 5661 | 18.0% | 838 | 13.4% | 5918 | 28.3% | <0.01 |
Treatment (baseline) | |||||||||
Psychiatry (yes/no) | 174,364 | 26.6% | 13,458 | 42.8% | 2558 | 40.9% | 12,465 | 59.5% | <0.01 |
Psychotherapy (yes/no) | 119,261 | 18.2% | 7057 | 22.5% | 1609 | 25.7% | 5930 | 28.3% | <0.01 |
Anxiolytics | 98,052 | 14.9% | 6486 | 20.6% | 1183 | 18.9% | 4371 | 20.9% | <0.01 |
Antipsychotics | 50,700 | 7.7% | 5239 | 16.7% | 1147 | 18.3% | 5470 | 26.1% | <0.01 |
Electroconvulsive therapy | 853 | 0.1% | 271 | 0.9% | 36 | 0.6% | 289 | 1.4% | <0.01 |
Transcranial magnetic stimulation | 781 | 0.1% | 48 | 0.2% | 11 | 0.2% | 34 | 0.2% | NS |
Suicidality (baseline) | |||||||||
Ideation | 8170 | 1.2% | 2629 | 8.4% | 1130 | 18.1% | 7053 | 33.7% | <0.01 |
Self‐harm attempt | 3261 | 0.5% | 991 | 3.2% | 558 | 8.9% | 2337 | 11.2% | <0.01 |
Acute care services | |||||||||
ED visits (all‐cause) | 275,047 | 41.9% | 22,799 | 72.5% | 4384 | 70.1% | 17,209 | 82.2% | <0.01 |
ED visit (psychiatric) | 14,723 | 2.2% | 6586 | 21.0% | 805 | 12.9% | 7861 | 37.5% | <0.01 |
Inpatient hospitalization (MDD) | 8801 | 1.3% | 2761 | 8.8% | 496 | 7.9% | 4287 | 20.5% | <0.01 |
Inpatient psychiatric facility (MDD) | 1506 | 0.2% | 596 | 1.9% | 84 | 1.3% | 911 | 4.4% | <0.01 |
Partial‐day hospitalization (MDD) | 508 | 0.1% | 252 | 0.8% | 8 | 0.1% | 147 | 0.7% | <0.01 |
Predicting Outcomes
The ROC curve for each predictive model derived from the validation sample is presented in Figure 2. The model predicting all‐cause ED visits had the lowest AUC (0.790). All other models had an AUC in excess of 0.87, with the models predicting suicide attempt (0.909), suicidal ideation (0.919), any suicidality (0.916), inpatient psychiatric facility admission (0.907), psychiatric ED visit (0.919), and partial‐day hospitalization (0.937) all in excess of 0.90. Table 3 lists the sensitivity, specificity, PPV, and negative predictive value (NPV) for each model at various percentile cut‐points of predicted risk.
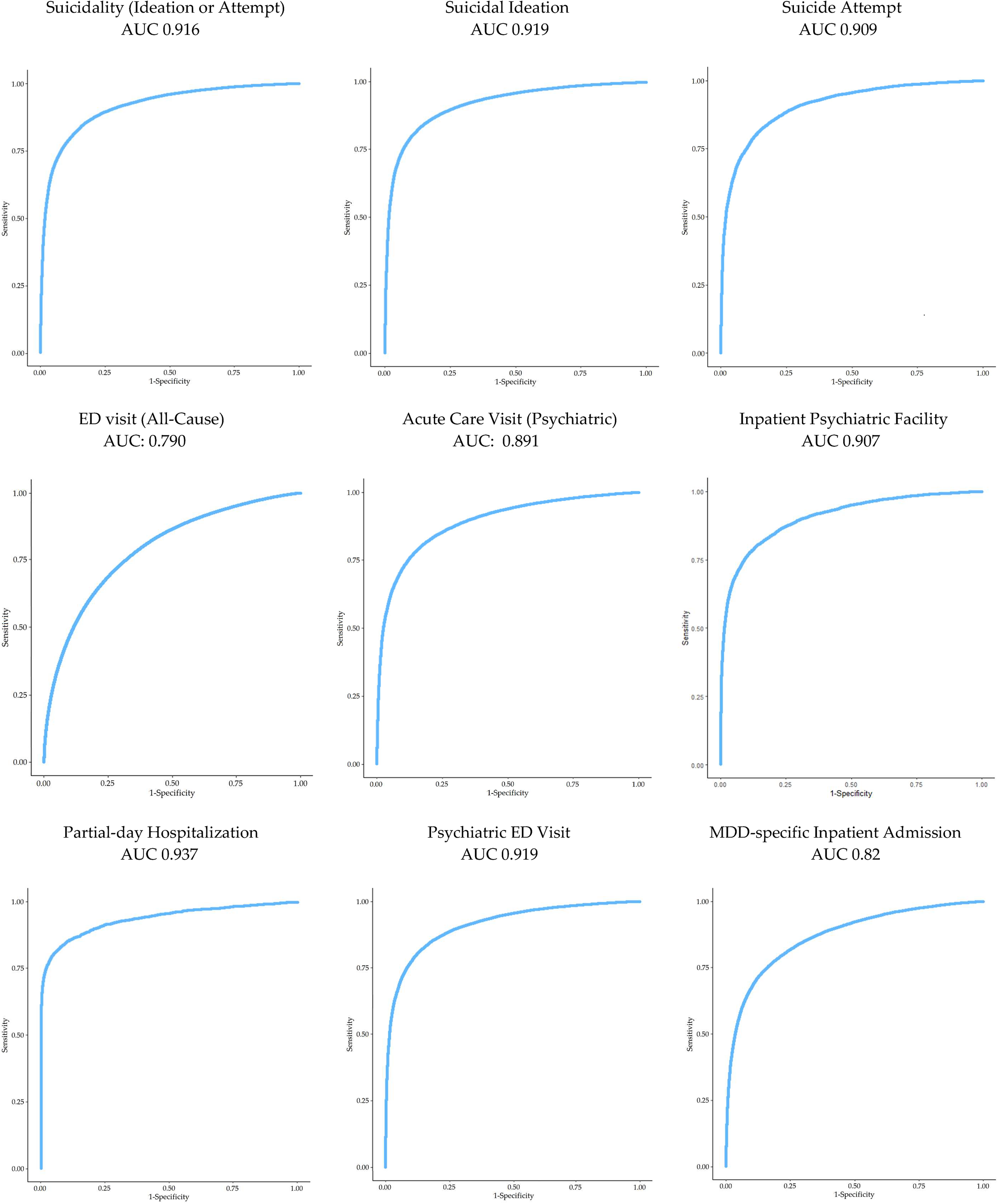
FIGURE 2. Receiver operator characteristic curves and area‐under‐the‐curve (AUC) for predicting outcomes, derived from the validation dataset.
Cutpoints (percentiles) | Suicide (ideation or attempt) | Suicide ideation | Suicide attempt | |||||||||
---|---|---|---|---|---|---|---|---|---|---|---|---|
Sensitivity | Specificity | PPV | NPV | Sensitivity | Specificity | PPV | NPV | Sensitivity | Specificity | PPV | NPV | |
99.5 | 1.4 | 100.0 | 99.5 | 64.6 | 1.5 | 100.0 | 98.8 | 68.8 | 4.3 | 100.0 | 96.9 | 89.2 |
99 | 2.8 | 100.0 | 99.8 | 64.9 | 3.1 | 100.0 | 98.7 | 69.2 | 8.3 | 99.9 | 94.3 | 89.6 |
95 | 13.7 | 99.9 | 98.3 | 67.5 | 15.5 | 99.8 | 97.9 | 72.0 | 37.6 | 99.1 | 84.9 | 92.6 |
85 | 40.1 | 99.0 | 95.8 | 74.8 | 45.0 | 98.8 | 94.7 | 79.6 | 72.0 | 92.3 | 54.2 | 96.3 |
75 | 63.3 | 96.4 | 90.8 | 82.5 | 69.2 | 95.4 | 87.4 | 87.1 | 83.6 | 82.5 | 37.7 | 97.5 |
50 | 90.2 | 72.6 | 64.7 | 93.0 | 91.6 | 69.3 | 57.8 | 94.7 | 94.6 | 55.8 | 21.4 | 98.8 |
Cutpoints (percentiles) | All‐cause ED visits | Psychiatric acute care services (Any) | Psychiatric inpatient facility | |||||||||
Sensitivity | Specificity | PPV | NPV | Sensitivity | Specificity | PPV | NPV | Sensitivity | Specificity | PPV | NPV | |
99.5 | 4.3 | 99.9 | 75.3 | 91.7 | 1.0 | 100.0 | 99.5 | 48.8 | 7.9 | 100.0 | 95.7 | 94.4 |
99 | 7.7 | 99.6 | 66.8 | 91.9 | 1.9 | 100.0 | 98.8 | 49.0 | 15.6 | 99.9 | 94.7 | 94.8 |
95 | 25.7 | 97 | 44.6 | 93.2 | 9.5 | 99.8 | 98.5 | 51.0 | 53.5 | 98.2 | 65.0 | 97.0 |
85 | 50.2 | 88.4 | 29 | 94.9 | 28.3 | 99.3 | 97.6 | 56.7 | 77.2 | 89.1 | 31.3 | 98.4 |
75 | 64.6 | 78.8 | 22.4 | 95.9 | 46.5 | 98.0 | 96.1 | 63.3 | 84.7 | 79.0 | 20.6 | 98.8 |
50 | 84.8 | 53.4 | 14.7 | 97.4 | 80.3 | 82.6 | 83.0 | 79.9 | 94.2 | 53.1 | 11.5 | 99.3 |
Cutpoints (percentiles) | Partial‐day hospitalizations | Psychiatric ED visits | MDD inpatient admission | |||||||||
Sensitivity | Specificity | PPV | NPV | Sensitivity | Specificity | PPV | NPV | Sensitivity | Specificity | PPV | NPV | |
99.5 | 17.4 | 100.0 | 99.0 | 97.6 | 1.3 | 100.0 | 99.4 | 62.3 | 1.91 | 99.98 | 97.74 | 74.80 |
99 | 34.8 | 100.0 | 99.1 | 98.1 | 2.6 | 100.0 | 99.2 | 62.6 | 3.77 | 99.95 | 96.52 | 75.15 |
95 | 77.1 | 97.1 | 43.9 | 99.3 | 12.9 | 99.9 | 98.5 | 65.1 | 18.30 | 99.58 | 93.72 | 78.02 |
85 | 86.4 | 87.1 | 16.4 | 99.5 | 38.0 | 99.2 | 96.8 | 72.3 | 48.78 | 96.64 | 83.29 | 84.60 |
75 | 90.7 | 77.0 | 10.3 | 99.6 | 60.4 | 96.9 | 92.3 | 79.9 | 67.88 | 89.79 | 69.53 | 89.06 |
50 | 95.5 | 51.5 | 5.4 | 99.7 | 88.8 | 74.1 | 67.8 | 91.5 | 87.66 | 63.06 | 44.90 | 93.70 |
DISCUSSION
Early identification of and intervention to support individuals at elevated risk of suicidality has been a public health priority for decades (18, 27, 28). Suicide is the 10th leading cause of death in the United States (29) and the rate of suicide has been steadily increasing, with age‐adjusted rates increasing from 10.5 per 100,000 in 1999 to 13.9 per 100,000 in 2019 (30), resulting in 47,511 suicide deaths in the United States (31). The prevalence of suicidal ideation and of suicide attempts is substantially higher than the rate of suicide. The 1‐year period prevalence rate of suicidal ideation among adults in the U.S. is estimated to be 4.8% and the 1‐year period prevalence of suicide attempts is 0.6% (32). These rates translate to suicidal ideation among 12.0 million and attempted suicides by 1.4 million adults, annually. Given that our study population is actively seeking care for MDD, the suicidality rate was substantially higher at 7.6 PkPPM, which roughly translates to 9.1 suicidal events per 100 persons per year. Our analytical models, each with an AUC in excess of 0.9, have the predictive accuracy to support real‐world implementation.
These results are consistent with those reported by others who also used advanced analytical methodologies to develop predictive models to identify individuals at risk for suicidal ideation and attempts at self‐harm. Simon et al. (19) developed a predictive model among nearly 3 million individuals in the U.S. to identify suicide attempts and deaths in the 90 days following an outpatient primary care or specialty mental health visit. Using a comprehensive set of variables derived from electronic health records characterizing up to 5 years of clinical history, this team developed predictive models with a c‐statistic of 0.851 (suicide attempts) and 0.861 (suicide death) following a mental health specialty visit and of 0.853 and 0.833 following a primary care visit. In another study to evaluate the predictive accuracy of suicide attempts, Barak‐Corren et al. (33) modeled risk factors derived from electronic health record data, among a population of individuals who sought care for any reason and reported an AUC of 0.73 and concluded that individual‐level risk prediction algorithms using real‐world health care data was feasible.
With AUCs in excess of 0.85, the models predicting inpatient hospitalizations accurately identify individuals with MDD at increased near‐term risk. A systematic literature review conducted by Prina et al. (10) reported that depressive symptoms are associated with elevated risk of all‐cause hospitalization, longer length of stay, and higher re‐admission risk. Further, a study by Voelker et al. (34) found that depression symptom severity is associated with increased risk of all‐cause and MDD‐related hospital encounters. A meta‐analysis by Zhou et al. (35) reported that predictive models that focused on risk of unplanned hospital readmissions had moderate discriminative ability, but were also found to have inconsistent performance, highlighting the need for rigorous validation of predictive models with moderate‐to‐high discriminative ability.
Frequently managed in primary care (36), early identification and management of MDD is essential (37). Though effective for many, the standard care model including both psychotherapy and pharmacotherapy is often associated with incomplete or inadequate treatment response (38, 39, 40). Comprehensive, team‐based care models are among the most effective interventions to support individuals with MDD (41, 42, 43); however, these programs are more complex and resource intensive care models to implement and sustain (44). By developing overall and outcome‐specific models, organizations considering an alternative care model for individuals with MDD can better target and prioritize the at‐risk populations, and with the full model results, can customize the selection of the at‐risk population by modifying the score cut‐point, to find a practical balance between identifying at risk population and the realistic number of individuals who can be contacted and enrolled in an intervention.
Implementing these models to identify and intervene on individuals at elevated risk may bring immediate benefits. At a national level, the incremental economic burden of MDD is substantial with significant use of inpatient and ED services attributable to MDD or to comorbidities among individuals with MDD (45, 46). More directly, a recent study by Chakravarthy et al. (47) reported that approximately 1.2% of all visits to the ED were coded for depression, with 40% resulting in hospital admission. Beiser et al., (48) reported an increased and independent risk of both ED visits and hospitalizations among those with depression, noting that the identification of high‐risk individuals can provide immediate value in the management of MDD. Complementing these findings, the current predictive model—with an AUC of 0.79—provides an alternative model for early identification of this at‐risk population.
Call to Action
Given the close collaboration required between insurance and provider organizations, there is substantial opportunity for collaboration in accountable care interventions. These, or related, algorithms should be automated and integrated into systems with administrative claims data or modified for use in E.H.R. systems to guide health systems, care providers, or depression care management programs in routing individuals with MDD to the most appropriate types of care at the appropriate frequencies to adequately manage their disease. The models developed in our research, along with those recently developed by others, report predictive accuracy (sensitivity and specificity) and efficiency (PPV) measures that will allow for the customized selection of score thresholds (cut‐points) that optimize the potential impact of an intervention with the resource constraints common to every program or intervention.
Strengths
Strengths of this study included the scale of the available dataset and representativeness of the population. Once the MDD eligibility criteria was applied, the dataset included over 5 million individuals. The dataset also included a wide variety of ages, geographic locations, and insurance types. The social determinants in this dataset were closely matched with those of the wider US population. Finally, this research developed an array of clinically‐relevant features to test in the predictive models, including changes in diagnosis, disease severity, healthcare resource use, and medication use.
Limitations
This study is subject to limitations. Administrative medical claims data are coded for reimbursement and may not accurately reflect clinical characteristics or healthcare resource utilization patterns. Administrative pharmacy claims data represent prescriptions that were paid for and retrieved but may not include all that were prescribed or represent a guarantee of ingestion. The dataset does not include eligibility and so complete capture of all healthcare utilization cannot be verified. The data may more closely approximate those available to an open healthcare system or provider group, rather than an insurance provider. MDD is under‐reported and under‐treated. It is likely that any effects noted underestimate the actual risk as not all individuals seek care, and healthcare providers do not universally screen for depressive symptoms. Similarly, suicidal ideation and behavior are likely under‐reported. These false negative outcomes will bias our results toward the null, reducing our ability to identify factors predictive of these outcomes. Finally, a key outcome measure, ED and inpatient hospitalization, is included as a negative outcome, but for some individuals these acute care services may be an essential source of support and may not be considered a negative outcome. Data do not allow us to fully understand the interaction and the potentially positive/preventive impact of the event.
CONCLUSION
The predictive models developed in this study accurately identified individuals at near‐term risk for suicidal ideation, suicide attempt, psychiatric inpatient hospitalization, and psychiatric ED visit. Ultimately, such models should be incorporated in routine practice to identify individuals at elevated risk for one or more of these high‐cost or high‐impact events and to intervene with a care model before the event occurs. The development and preliminary validation of these analytical models in the current study represents the first step in the research and implementation process. Given the diverse study population, future research should consider variability in model performance across major population subgroups, beginning with those individuals with and without well‐documented risk factors, such as history of suicidality, substance use, etc. Future research into the utility and value of these models would also benefit from further verification of the predictive accuracy in one or more independent data sources, preferably one that derives from an accountable care or provider organization with an intervention program or care model designed to engage and support individuals with MDD.
1
2
3
4
5
6
7
8
9
10
11
12
13
14
15
16
17
18
19
20
21
22
23
24
25
26
27
28
29
30
31
32
33
34
35
36
37
38
39
40
41
42
43
44
45
46
47
48